What is Augmented Network Automation?
An Augmented Network Automation Platform is a sophisticated management system for complex mobile networks in the telecommunications industry, built on the established framework of a Self-Organizing Network (SON). Using machine-learning and AI to automate network management, an Augmented Network Automation platform meets the growing demands of network complexity and changing scale with a flexible, automated system. Automated tasks include:
- Using self-healing and reinforced learning to detect network anomalies
- Automatically conduct root cause analysis
- Make corrections and changes
The platform also enables a high degree of customization so mobile network operators (MNOs) can create their own use-case based applications. Benefits of this management platform ultimately include simplified network management complexity and improved end-user customer satisfaction, with a high rate (up to 20%) of deployment and optimization of 4G/5G wireless networks and services, and improved subscriber quality of experience (QoE) with a reduced drop call rate of up to 30%.
The Evolution from SON to Augmented Network Automation
SON technology is a widely established management system for mobile network operators (MNOs), varying in complexity from the simple, such as managing power for cell sites by load balancing, to the more complex (covering transport, core, and providing automation). But coping with the complexity of multiple radio access technologies (RATs) and multiple radio access network (RAN) vendors requires a sophisticated, automated solution, especially as new frequency bands and new technologies are introduced (such as 5G or open RAN) or retired (such as 3G).
Other needs for an end-to-end automation solution are driven by increases in user service and bandwidth demands, while mobility domains such as packet core, policy, and backhaul are all affected. Fortunately, because of progress in advanced machine-learning algorithms, it’s now possible to enhance existing SON solutions.
Machine learning algorithms can be used where there is a need to understand patterns in the network. These algorithms can detect cells with suboptimal performance, which might not be identified using classic detection approaches.
To become proactive, prediction-based machine learning models are used to help foresee network behavior and performance. These machine learning models use cell radio configuration as well as historical performance of the cell and co-related cells to provide accurate predictions. Knowing about network degradation in advance and fixing the issue before performance degradation occurs can greatly improve the user experience. In addition, the predictive capabilities of machine learning can support the need to know about the consequences of each corrective action a SON may execute. Machine learning optimized networks is faster and more effective than classic algorithms; it can select the optimal choice for the required impact on network performance.
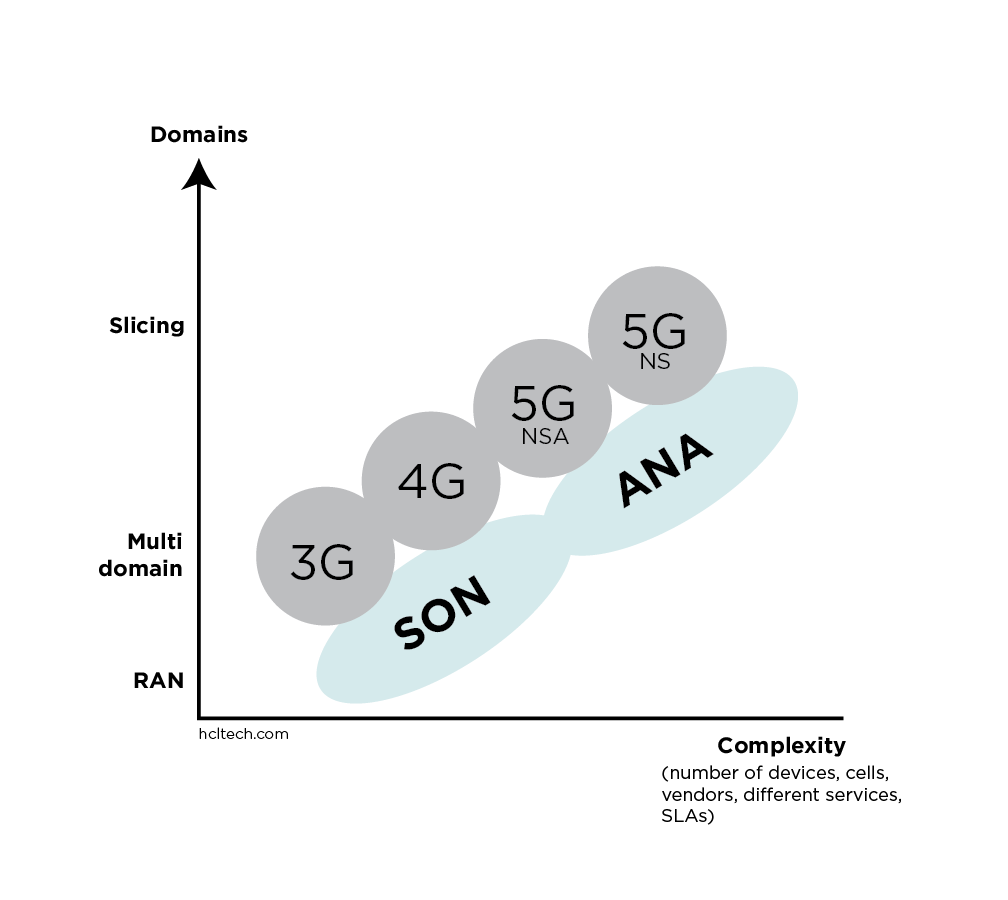
An advanced SON solution moves from the previously reactive solution to a proactive one, while simplifying and automating network operation practices. An Augmented Network Automation platform accomplishes this, providing the benefits MNOs require. The platform collects and processes the data, as well as performing proactive multi-domain action using machine learning (ML) analytics capabilities.
The platform reduces both man-hours and operational errors for processes such as remote configuration of new base station equipment, new services enablement, such as narrowband-Internet of Things (NB-IoT), and parameter audits. This solution processes vast amounts of data in real time to detect issues from subscribers, multiple services, multiple domains of network slices and proactively perform corrective action.
The evolution from traditional SON to the intelligent and autonomous Augmented Network Automation platform ultimately reduces the burden on operations and engineering teams. Benefits of the platform include:
- Easier network upgrades
- Fewer dropped calls
- Higher data rates
- Less network congestion
- Increased customer satisfaction
Augmented Network Automation Platform Overview
An Augmented Network Automation system employs machine learning and sophisticated algorithms to optimize across multiple tunable parameters, and carries out closed loop (autonomous) self-healing so that the network is monitored, analyzed, and adjusted without taking valuable resources from the operator. This is especially important as networks scale to greater complexities, which means larger datasets and a greater volume and variety of data, and ultimately a greater difficulty in extracting actionable insights for network performance from that data. Machine learning automates this difficult process.
A machine learning analytics cycle begins by monitoring target KPIs (for example, throughout or dropped call rate). Then, if anomalies are detected, a root cause analysis is run to identify the culprit. Sometimes load balancing RAN will solve the issue, but in other cases the Augmented Network Automation Platform may need to instruct the mobile core to the temporarily reduce the maximum bit rate for a user, or a group of users, or even a specific type of service.
Network Automation in Telecom
Many MNOs have both 4G and 5G technologies deployed in their networks. Increased subscriber quality of experience (QoE) demands (such as video streaming applications) require flexibility, scalability, and automation that meets the increased complexity and diversity of subscribers. The need for additional functions such as geolocation, crowdsourcing, big data analytics, and the Internet of Things (IoT) further increases network complexity.
Augmented Network Automation goes beyond the constraints of traditional SON systems in the following ways:
- Self-healing: networks are monitored, analyzed, and maintained in a closed-loop (autonomous) environment
- Open platform for innovation: The open platform supports ORAN and enables service providers and partners to develop and quickly deploy custom applications according to specific use cases
- Flexibility in network operation: the Augmented Network Automation Platform runs in both the operator’s cloud or in the public cloud, surpasses traditional SON limitations of running on dedicated servers in the operator’s network. Easily scale networks with a cloud-based platform that enables flexible deployments (on-premises, hybrid, and public cloud).
The telecom industry has been slow to adopt total machine learning, and this is largely due to overpromises made by vendors early on. However, the technology has caught up with the theory, and measureable benefits shown by the Augmented Network Automation platform combined with fast-increasing technologies mean that AI and machine learning systems are ready to be implemented now.
AI Machine Learning Automation
Augmented Network Automation minimizes the impact of rapid changes in the network, which keeps performance from degrading, by proactively identifying root causes that adversely affect the network and acting to prevent them before they occur. This is accomplished by:
- Detecting the behavior patterns hidden in the mass of measurements at the network level
- Using the clusterization of similar network cells to identify cells with suboptimal performance
- Analyzing the root causes on these cells to optimize performance
Augmented Network Automation automatically predicts, configures, and optimizes cellular networks, leveraging self-healing and reinforced learning techniques. Using these methods, human intervention is reduced and errors are minimized.
Why Use Machine Learning in Network Management
Closed-loop automation across network layers allows MNOs to get predictive optimization and hands-free, 24/7 network configuration that automatically corrects any anomalies. This means a faster time to market for new services across 5G networks.
An Augmented Network Automation platform is itself an open platform for third-party applications, whether developed by the operator or by a third party. This is a significant change from traditional SON systems, which were difficult for operators to develop their own use cases. Augmented Network Automation systems have a low-code development environment, with an intuitive user interface, so engineers lacking the coding skills required in traditional SON systems can now build their own use cases without needing vendor support—and at a much faster time to market (weeks vs months).
Augmented Network Automation Use Cases
Mobile network operators can use an Augmented Network Automation platform to see competitive advantages by increasing operational efficiency. Augmented Network Automation use cases include:
- Faster rollouts of 5G apps: Augmented Network Automation automates 5G network deployment and ensures a fast and efficient rollout by detecting potential issues and correcting them before implementation. This is useful not only for 5G deployment and optimization, but also interaction between 4G and 5G apps.
- Cloud based services: Augmented Network Automation can function as a service on its own, which is especially appealing to private LTE/CBRS and enterprise customers.
- Analytics: third party data source integration, machine learning and AI, subscriber-centric analysis, data anaclitic functions
- ORAN: Cluster SON for open disaggregated and virtualized RAN, real-time subscriber centric optimization, and visibility and actions at the subscriber level.
- Multidomain service optimization: end to end slice SLA management and transport-aware SON
Benefits of Augmented Network Automation
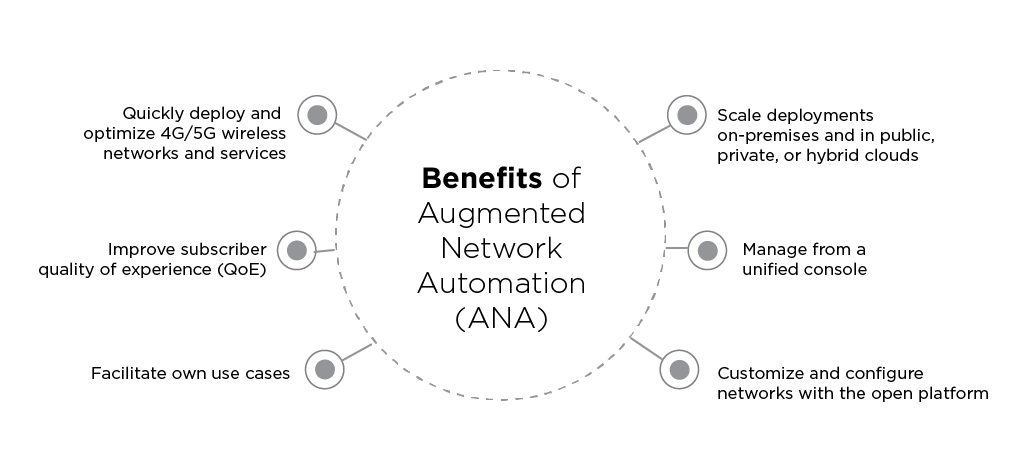
A robust Augmented Network Automation platform offers customization and operational efficiency from a single point of management, automating key areas of network management and freeing up MNO resources. In general, Augmented Network Automation aims to:
- Quickly deploy and optimize 4G/5G wireless networks and services, which can be seen by an increase in data capacity of up to 20%
- Improve subscriber quality of experience (QoE), which can be realized by reducing drop-call rates by up to 30%
- Facilitate own use cases with a low-code environment and an intuitive user, meeting key user quality indicators (KQls)
- Scale deployments on-premises and in public, private, or hybrid clouds
- Manage from a unified console the different layers, technologies, vendors and architecture, whether it’s ORAN or classic Element Management System (EMS) networks. This results in efficient network scalability and improved productivity to reduce Total Cost of Ownership (TCO). This network flexibility reduces operational risk as MNOs no longer require vendor lock-in for network solutions.
- Customize and configure networks with the open platform according to individual network operator requirements and plans, resulting in more choice with less